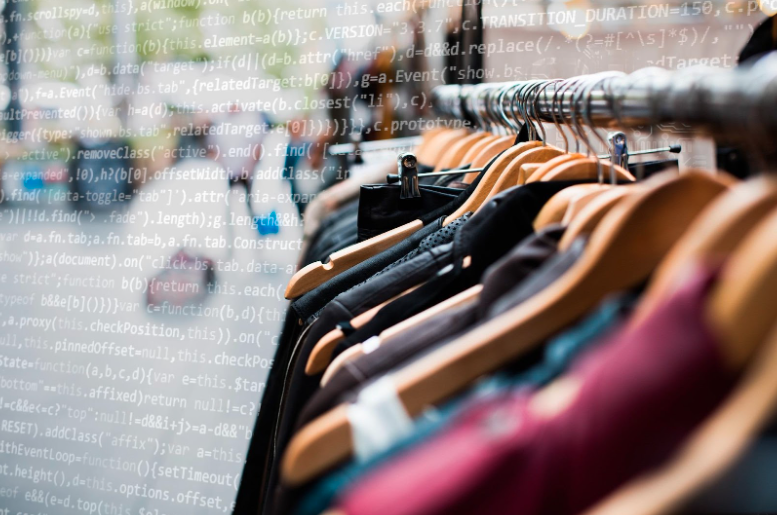
Fashion trends can change seemingly overnight, and companies and brands sometimes find it difficult to design the right styles and then market them to the right people, on the right platforms, at the right time. With advancements in artificial intelligence, big data analysis, and machine learning, however, businesses can now automate and perform many of these important tasks with a surprisingly high level of accuracy. One such company is Stitch Fix, which was founded in 2011 to better understand consumers’ personal styles and help them easily discover clothes that fit their tastes, needs, and lifestyle.
Since its inception, Stitch Fix has been simplifying the discovery of fashion and styles that resonate with users. By using AI and effectively analyzing and decoding vast amounts of user data, Stitch Fix now serves over 3 million people across the U.S. The service has been featured and field-tested by numerous fashion and lifestyle outlets and can provide unique style and fashion recommendations for every imaginable scenario.
Millions have signed up for Stitch Fix, sharing information like size, budget, style and fit preferences, and what type of occasion they’re shopping for, be it attire for casual Fridays to what to sport while backpacking in Paris. This provides 85-plus data points, helping expert stylists and algorithms work in sync to curate a “Fix” — five articles of clothing or accessories delivered to the client’s home where they can try them on and evaluate each piece, keep what they like and send back what they don’t.
Understanding the unique styles of individuals on such a large scale — especially since everyone’s tastes and preferences are deeply personal, nuanced, and difficult to quantify — is no small feat. Using a proprietary algorithm called “Latent Style,” Stitch Fix extracts insights from myriad places including billions of ratings collected through Style Shuffle — the platform’s Tinder-esque like/dislike game for rating accessories and pieces of clothing — the client’s own Style Profile, interactions with a personal stylist, Fix request notes (which number an impressive hundreds of thousands monthly), and the checkout feedback left by 85% of clients.
Latent Style is the cornerstone of Stitch Fix’s innovation, and it enables the personal styling service to forecast not just the items that users will like, but what kinds of recommendations to serve them and even the emails to curate for them.
Stitch Fix in the Evolving Style Matrix
Early on, Stitch Fix made use of both human stylists and algorithms to create the perfectly tailored Fixes — five hand-picked pieces sent right to a client’s doorstep. It remains the company’s core model.
Soon after, Stitch Fix introduced Style Shuffle, a “Tinder for clothes” game played by around 1 million clients monthly, highlighting preferences and personal style with a thumbs-up or thumbs-down on items. Soon after it was introduced, Style Shuffle proved to be a hit with customer engagement while helping stylists and algorithms better understand clients’ preferences. And with Style Shuffle recently hitting an astounding 10 billion interactions since its introduction in March 2018, Stitch Fix is building the most extensive and data-driven understanding of personal style in the world.
Armed with this influx of data, Latent Style could extract even more meaningful insights. By leveraging ratings from Style Shuffle to understand user preferences on a deeper level, Latent Style could, for example, infer that a user who likes a certain kind of top would also appreciate particular bottoms that correlated positively with those kinds of tops for users who have similar taste and style profiles.
It enables Stitch Fix to understand who likes what, while providing personalized recommendations for users who haven’t yet played Style Shuffle simply because they have a Style Profile similar to that of someone who has played it. And clients are having a blast doing it.
The impact of this AI-driven approach extends beyond immediate interactions. The algorithm assists with curating future clothing boxes for clients, ensuring a continuous alignment with customer preferences. Style Shuffle ratings are combined with feedback on received items, which allows Stitch Fix to refine its understanding of what customers really want.
Style Shuffle is also designed to align customers’ descriptions of their style preferences with the company’s understanding of that input. Thanks to the simplicity of thumbs-up/thumbs-down ratings, the ambiguity of descriptive terms is eliminated, creating a shared language for both users and the AI. Stitch Fix’s stylists can access what their clients awarded a thumbs-up — or a thumbs-down — contributing to a richer understanding of style preferences. The game’s potential extends to testing new items from in-house brands before production, showcasing the game’s versatility in informing various aspects of the business. Over time, Style Shuffle feedback trends displaying what a client loves — and what they don’t — leads to an optimization of increased success rates and happy clients who are getting more clothes they love at the exact moment they need them. Talk about tailor-made recommendations.
Pandemic-Era Fashion Findings
Why does it work so well? Adaptability, for one thing, says Manju Thomas, SVP engineering and data service at Stitch Fix.
“Our depth of knowledge uniquely positions us to adapt to rapidly changing client trends as they evolve and work more productively with our brands to source merchandise our clients love,” Thomas told the Insider Intelligence website. “For example, during the first few months of the pandemic, we saw a tenfold increase in requests for ‘working from home’ and a shift out of formal workwear and into more casual, everyday styles. We took a proactive approach to adjusting our assortment, refocusing our spring and summer assortment to support a more versatile life while cutting workwear down by 10%.”
Based on research, over half of Stitch Fix’s clients said that their preferred looks and style changed during the COVID-19 pandemic. About two-thirds of survey respondents said that they were likely to replace the clothes in their wardrobes, with a desire to keep up with new styles and trends (36%), needing a different size (32%), needing clothes for social activities (27%), needing clothes for specific events (19%), and going back to the workplace (17%) being the leading reasons for wardrobe overhauls. Almost one-third of respondents said they would prefer a 10% pay cut over having to get dressed up for work every day, and an overwhelming 77% said that they had sworn off at least some form of business attire for good.
Thomas told Intelligence Insider that Stitch Fix can also see what factors are influencing clients’ shopping habits. “Each year, we combine client data along with key insights from our stylists and merchants to develop our Style Forecast. Our 2023 report found that nearly three-quarters of our clients believe the economy will impact the way they shop for clothes this year, and 80% of clients want to be more thoughtful when shopping for clothes.
“This sentiment is fueling the rise of what we call the ‘intentional wardrobe,’ which means more people are investing in high-quality, versatile clothing. In addition, the concept of cost per wear is becoming very relevant. With this intelligence, we can anticipate increased demand for trend-proof basics or foundational pieces in neutral colors and prints.”
Improving the Stitch Fix Algorithm
As users’ style evolves, so too does the Stitch Fix algo.
The Latent Style algorithm continuously learns and adapts based on user feedback and behavior. As customers receive and provide feedback on their personalized Fixes, the algorithm refines its understanding of their style, ensuring that each subsequent fix is a closer match to the customer’s preferences.
More than being just a fun game to drive engagement, Style Shuffle has helped Stitch Fix learn a great deal about their user’s styles, what they are most likely to want to wear and buy, and what to recommend to them. Also, whenever new items are added to Style Shuffle and customers rate them, the map of possible combinations and style options expands.
Furthermore, by smartly including items that will most likely receive a thumbs-up (and therefore encourage users to continue playing) alongside items that Stitch Fix wants more data on and restricting how much users can play and how many items they can rate at a time, the company can generate a vast amount of useful intel on what sells and why, and what kinds of items to show to whom, and when.
The role of AI throughout this process is pivotal. As Latent Style learns by processing a wide range of data points such as customer feedback, current trends, fashion blogs, and even fabric textures, it can predict not only what items an individual might like, but also how those items will fit into their existing wardrobe. Stitch Fix uses AI to take things a step further by predicting sizes accurately, accounting for factors such as brand-specific sizing variations, fabric elasticity, and the individual’s previous fit feedback.
Beyond Style Shuffle, Stitch Fix has algo-dedicated teams for every department.With all of these offerings, Stitch Fix is spearheading a new era of innovation and personalization for the fashion industry. As AI technologies improve, possibilities abound for enhancing offerings, capturing new markets, and redefining the fashion landscape. Brands and businesses that embrace these opportunities will be poised to thrive, and the Stitch Fix blueprints for data-driven analysis, recommendations, and optimization stand as effective starting points for industry players to tackle the inevitable change that will come their way.